Comparing Wanted vs Accessed Services in Data Analysis

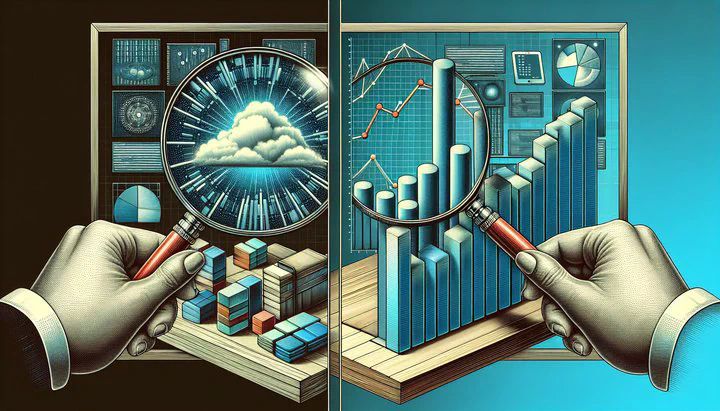
Comparing Wanted vs Accessed Services in Data Analysis
Understanding the Difference
In data analysis, it’s crucial to understand the difference between wanted services—the tools and resources you plan to use—and accessed services—the ones you actually end up using. This distinction can significantly impact your project’s success.
Imagine planning to use a high-powered statistical software that promises deep insights. This is a wanted service because you believe it will deliver the best results. However, due to budget constraints or complexity, you may end up using a simpler tool instead, your accessed service.
Understanding why there’s a gap between these two can help you optimize your data analysis process. By analyzing wanted versus accessed services, you can align your expectations with reality, ensuring you use the best tools available for your projects. This alignment not only makes your projects more efficient but also more effective.
Why the Gap Exists
When you start comparing wanted vs accessed services in data analysis, you’ll often notice a gap between what you hope to use and what you actually use. This gap can be frustrating, but understanding why it happens can help you address it.
One of the main reasons for this gap is budget limitations. You might have your eyes set on advanced software or premium tools, but sometimes, the cost is just too high. Many data analysis tools come with hefty price tags, and not every project has the funds to cover them. This forces you to choose more affordable, and sometimes less effective, alternatives.
Another factor is the lack of access to certain tools. Even if you can afford them, some tools might not be available due to licensing restrictions or institutional policies. For instance, a university might only provide certain software to specific departments, leaving you without the tools you wanted.
Unanticipated changes in project needs can also lead to a gap. As projects evolve, the tools you initially wanted might no longer fit the new direction. You might discover halfway through your analysis that a different service is better suited for the data you’re working with, which means reassessing your needs and accessing different tools.
For example, imagine a research team planning to use a cutting-edge data visualization tool. Midway through their project, they discover that the tool’s learning curve is too steep for their timeline. Instead, they opt for a simpler, more accessible alternative. By comparing needed vs used services in data analysis, they adapt to their constraints and keep their project on track.
Understanding these reasons is the first step to minimizing the gap. By being aware of budget, access, and evolving needs, you can better prepare to align your desired and utilized services in data analysis, leading to more successful outcomes.
Impact on Data Projects
When considering the impact of wanted vs accessed services on your data projects, it’s vital to recognize how these differences can affect your outcomes. If you can’t use the tools you planned for, your project might not turn out as you’d hoped.
Imagine you’ve planned an analysis with a powerful statistical software package. This tool was part of your wanted services because it could provide deep insights and detailed visualizations. However, due to budget cuts, you end up using a basic spreadsheet program instead. This switch to accessed services can significantly limit your project’s depth and scope. The advanced analyses you hoped to run might not be possible, leading to less comprehensive results.
The gap between comparing desired and utilized services in data analysis can also cause delays. Without the right tools, you might spend more time trying to achieve the same results, which can slow down your entire project timeline. This delay can be frustrating, especially if you’re working under tight deadlines or when a project’s success relies on timely completion.
Moreover, the wanted vs accessed services impact extends to data quality. Using inadequate tools may lead to errors or inconsistencies in your analysis. This can affect the reliability of your findings, making it harder to draw accurate conclusions or make informed decisions. On the other hand, when you successfully align your wanted services with what you access, it enhances the overall quality and credibility of your work.
By analyzing wanted versus accessed services, you can identify gaps and find solutions to bridge them. This strategic approach not only improves the efficiency of your projects but also ensures you deliver high-quality results. When you have access to the right tools, your data projects become more robust, allowing you to make sound, evidence-based insights.
Strategies to Align Services
Aligning your wanted and accessed services in data analysis is essential for achieving the best results in your projects. Here are some strategies to help you close the gap and make the most out of the resources available to you.
First, prioritize essential tools. Determine which services are absolutely necessary for your project’s success. Make a list of the tools and resources that will have the biggest impact on your analysis. By focusing on the most crucial services, you can allocate your budget more effectively and ensure you have access to the key tools you need.
Next, consider seeking alternative resources. If budget constraints prevent you from accessing certain wanted services, look for free or open-source alternatives. For example, if a commercial statistical software is too expensive, explore free tools like R or Python, which offer robust data analysis capabilities. Many of these tools have active user communities and plenty of online tutorials, making it easier to learn and implement them in your projects.
Another strategy is to negotiate service access. If you’re part of an organization, see if there are opportunities to share resources with other departments or teams. Sometimes, pooling resources can make it easier to access desired services. You might also negotiate with service providers for academic or bulk discounts, which can make expensive tools more affordable.
Effective project planning and resource allocation are also key to aligning services. Before starting a project, take the time to carefully plan your needs and assess what you can realistically access. This involves setting clear goals and identifying potential obstacles early on. By having a well-thought-out plan, you can make informed decisions about which services to pursue and how to adjust if circumstances change.
Finally, stay informed about new tools and technologies. The world of data analysis is constantly evolving, and new solutions are regularly emerging. By keeping up with industry trends and participating in professional communities, you can discover new resources that might better fit your needs or budget.
By applying these strategies, you can better align your comparing desired and utilized services in data analysis, ensuring that your projects are both efficient and effective. This alignment not only optimizes your analysis process but also enhances the quality of your results, allowing you to achieve your data-driven goals with confidence.
Case Studies and Examples
Looking at real-world examples can help us see how aligning wanted and accessed services in data analysis can lead to successful outcomes. Let’s explore some case studies where data analysts have effectively managed these challenges.
Case Study 1: Academic Research Team
A university research team wanted to use a premium data visualization tool to present their findings. This tool was part of their wanted services because of its advanced features and stunning visuals. However, the team faced budget constraints and couldn’t afford the high licensing fees. Instead, they opted for a free, open-source tool, learning its features through online tutorials and community forums. By comparing needed vs used services in data analysis, they successfully adapted to their circumstances. The result was a visually compelling presentation that effectively communicated their research findings, demonstrating that with creativity and flexibility, even budget limitations can be overcome.
Case Study 2: Start-Up Data Analysis
A start-up company needed robust statistical software for a market analysis project. Initially, they wanted a top-tier commercial package known for its comprehensive analytics capabilities. However, they quickly realized the cost was prohibitive. Instead of compromising on quality, they explored alternatives and found a cost-effective solution by utilizing a combination of R and Python. These tools, which are highly regarded in the data community, offered the functionality they needed without breaking the bank. By analyzing wanted versus accessed services, the start-up not only completed their project successfully but also gained valuable skills in open-source software.
Case Study 3: Government Health Department
A health department aimed to analyze a large dataset to improve public health strategies. Their wanted services included a powerful cloud-based data platform for storage and processing. Unfortunately, due to data privacy regulations and security concerns, they couldn’t access the desired cloud services. The team adapted by setting up a secure in-house data processing environment. By negotiating access to necessary hardware and software within their organization, they maintained control over their data while achieving their project goals. This experience showed that even when faced with strict regulations, aligning desired and utilized services in data analysis is possible with strategic planning.
These examples highlight how different teams have navigated the challenges of aligning wanted vs accessed services impact. They illustrate that with the right strategies, it’s possible to overcome obstacles such as budget restrictions, access issues, and regulatory constraints. By learning from these case studies, you can apply similar approaches to your own data projects, ensuring that you use the best available resources to achieve your objectives.
Conclusion and Call to Action
By understanding and bridging the gap between wanted and accessed services in data analysis, you can significantly improve the efficiency and effectiveness of your projects. Remember to prioritize essential tools, explore alternative resources, and plan strategically. Stay informed about new technologies and engage with professional communities. These steps will help you align your resources with your project goals, leading to more successful outcomes. Now, take what you’ve learned and apply these strategies to your own data analysis projects, ensuring you achieve the best possible results.