Impact of Low Alpha Years on Data Analysis

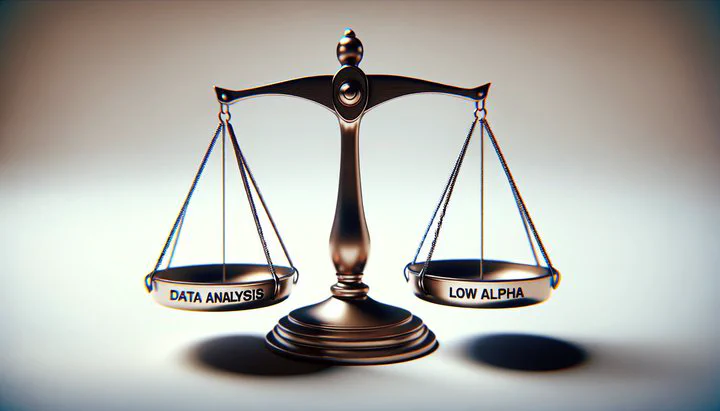
Understanding Low Alpha in Statistical Contexts
Have you ever wondered why scientists are sometimes extra cautious when interpreting their results? This is where low alpha years come into play. In the world of statistics, alpha is like a safety net – the lower it is, the more careful you are before declaring something a success. It often represents the probability of making a Type I error, where a true null hypothesis is incorrectly rejected. But what happens when we talk about low alpha years? These are periods where the alpha level is set very low, meaning we’re extremely cautious about making errors. Understanding the impact of low alpha years on data analysis is crucial because it influences how we interpret and trust our data.
Low alpha years matter because they change how we handle statistical tests. A low alpha level means that we’re setting a high bar for saying something is statistically significant. This can be important in fields like medicine or environmental science, where being wrong could have serious consequences. For example, if a new drug is being tested, a low alpha level might be used to ensure that any claims of effectiveness are highly reliable, minimizing the chance of false positives.
In data analysis, recognizing when you are in a low alpha year can help you better understand the results of your statistical tests. Imagine you’re reviewing a study and notice the researchers used a very low alpha. This tells you they were being extra careful, which might affect how you interpret the results. For instance, you might encounter fewer significant findings, but those you do find are more trustworthy. Knowing this helps you make informed decisions about the data.
Examples of situations where low alpha is encountered include regulatory settings or when dealing with high-stakes decisions. In such environments, setting a low alpha minimizes the risk of making false claims. However, it also means you might miss some true effects because the bar is set so high. Understanding this balance is key to effective data analysis. By grasping the significance of low alpha in these contexts, you can better navigate the challenges and make more informed interpretations of your data.
Effects of Low Alpha on Data Interpretation
When working with data, it’s important to understand how a low alpha level can affect your interpretations. The effect of low alpha on data interpretation is significant because it can lead to both cautious conclusions and potential misunderstandings. In a low alpha year, you’re asking for stronger evidence before declaring a result significant. This cautious approach helps prevent mistakes but can also make it harder to detect real effects.
Think of it like setting a very high bar for winning a game; only the most outstanding players can score, meaning you might miss out on recognizing some good players. One challenge analysts face with a low alpha is the risk of overlooking true findings. Because the threshold for significance is set very high, some actual effects might not meet the stringent criteria and could be dismissed as insignificant. This situation can lead to the belief that nothing is happening when, in fact, important patterns or relationships do exist.
To mitigate these challenges, it’s essential to use strategies that account for low alpha’s impact. Consider using a bigger team to increase your chances of scoring, similar to increasing your sample size in a study. Performing a power analysis before starting your study helps determine the sample size needed to detect meaningful effects, even with a low alpha. Additionally, using confidence intervals alongside p-values can provide a fuller picture of your results, helping you understand the range within which the true effect might lie.
Another strategy is to consider the context of your analysis carefully. Ask yourself why a low alpha was chosen and what the potential consequences might be. This understanding can guide how you interpret findings and communicate them to others. By recognizing that a low alpha means more caution in declaring significance, you can adjust your expectations and interpretations accordingly, ensuring that your conclusions are both cautious and well-supported.
Understanding the impact of low alpha years on data analysis empowers you to make informed decisions. By being aware of the possible misinterpretations and having strategies to address them, you can navigate the complexities of data analysis with a clearer, more accurate perspective.
Role and Consequences of Low Alpha in Statistical Analysis
Understanding the role of low alpha in statistical analysis is crucial for anyone involved in data-driven decision-making. In statistical terms, alpha is the threshold for deciding whether an effect or relationship is statistically significant. A low alpha acts like a tight filter, letting only the strongest signals through. But sometimes, important signals might be too weak to pass through, leading to missed opportunities. This cautious approach can help avoid mistakes, especially in critical fields like medicine or policy-making.
However, setting a low alpha also has its consequences on data evaluation. One major consequence is that it becomes more difficult to find statistically significant results. This might lead you to conclude that there are no meaningful effects when, in fact, they exist but are just hard to detect due to the stringent criteria. For example, in environmental studies, a low alpha might lead researchers to overlook subtle but important climate changes that don’t meet the high threshold of significance.
The impact of low alpha years on data analysis is also felt in decision-making processes. In real life, setting the bar too high could mean missing out on important clues, like ignoring a whisper that warns of danger. In fields where decisions must be made based on statistical evidence, a low alpha could mean missing out on opportunities to act on real effects. Imagine a scenario in which a new public health policy is being evaluated. If the alpha level is set too low, policymakers might not see the policy’s benefits and fail to implement changes that could improve public health outcomes.
To effectively handle the role and consequences of low alpha in your analysis, it’s important to plan your studies carefully. Conducting a power analysis can help ensure that your study is designed to detect effects even with a low alpha. Additionally, consider using other statistical tools like Bayesian analysis, which can provide different insights into your data.
By being aware of the impact of low alpha years on data analysis, you can better navigate the challenges they present. Always question why a low alpha was chosen and what this means for your findings and decisions. With this understanding, you can balance caution with the need to detect important effects, ensuring your analyses lead to well-informed and effective decisions.
To explore more about low alpha and other statistical concepts, visit StatisticalExplorer and try applying these ideas in your analyses. Happy exploring!