How to Interpret Inter Rater Reliability

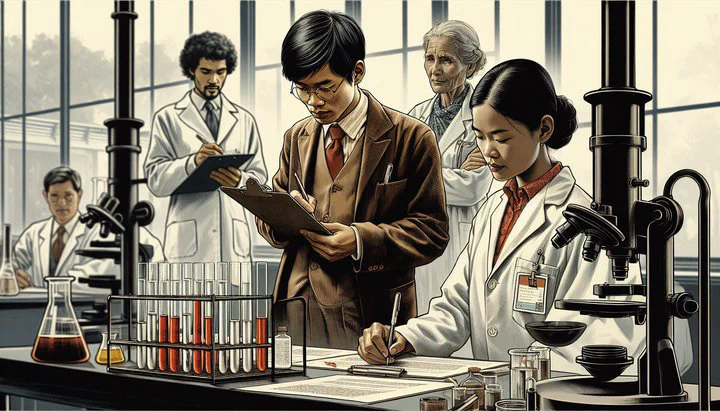
How to Interpret Inter Rater Reliability
When conducting research, ensuring that your data is consistent and reliable is essential for drawing accurate conclusions. One way to achieve this is by using inter rater reliability. But what exactly is inter rater reliability? Simply put, it is a measure of how much agreement or consistency there is between different people (or raters) who are observing or rating the same phenomenon. This concept is important because it helps researchers understand how much of the data variation is due to differences in raters rather than differences in the subjects being measured.
Inter rater reliability is particularly important in fields such as psychology, education, and social sciences, where subjective judgments can vary widely. For instance, if multiple teachers are grading the same essay, inter rater reliability ensures that the grades are consistent, no matter who grades the essay. Without it, results could be unreliable, leading to incorrect conclusions.
There are several ways to measure inter rater reliability, each with its own strengths. Some common methods include Cohen’s Kappa, which is used for categorical ratings, and the Intra Class Correlation Coefficient (ICC), which is used for continuous ratings and is particularly useful when more than two raters are involved. These methods help in quantifying the level of agreement among raters, providing a statistical measure that can be interpreted to ensure consistency.
Understanding and measuring inter rater reliability is essential for producing valid and reliable research findings. By ensuring that multiple observers or raters produce consistent results, researchers can confidently interpret their data. In the following sections, we will explore how to interpret inter rater reliability using the Intra Class Correlation Coefficient and other methods to ensure accurate and reliable research outcomes.
Using the Intra Class Correlation Coefficient
When it comes to understanding inter rater reliability, the Intra Class Correlation Coefficient (ICC) is a powerful tool. The ICC helps you interpret inter rater reliability by measuring the degree of agreement or consistency among raters. It’s particularly useful when you have more than two raters and the data is continuous. But how exactly do you use the ICC to assess inter rater reliability?
Think of ICC like a referee in a sports game ensuring that all judges score a performance consistently. The ICC compares how much raters agree with each other to how much they differ overall. There are different types of ICC, each suited for specific study designs. For example, the ICC(1,1) might be used when each subject is rated by a different set of raters, while the ICC(2,1) is used when all subjects are rated by the same set of raters. Choosing the right type of ICC is crucial for accurate interpretation.
To interpret inter rater reliability using the Intra Class Correlation Coefficient, you’ll look at the ICC value, which ranges from 0 to 1. A value closer to 1 indicates a high degree of agreement among raters, which means the ratings are consistent. On the other hand, a value closer to 0 suggests little to no agreement, indicating that the ratings are inconsistent. Typically, an ICC above 0.75 is considered excellent, between 0.60 and 0.75 is good, between 0.40 and 0.60 is fair, and below 0.40 is poor.
Using the ICC to interpret inter rater reliability provides a robust way to ensure your data’s reliability. By understanding the level of agreement among raters, you can make informed decisions about the quality of your data. In the next section, we will guide you on how to assess whether there is inter rater reliability in your dataset, ensuring your research conclusions are based on reliable data.
Assessing Inter Rater Reliability
Assessing whether there is inter rater reliability in your dataset is a crucial step in ensuring your research findings are valid and trustworthy. This process involves several steps, from preparing your data to choosing the right statistical tools for analysis. Let’s walk through how you can confidently assess inter rater reliability in your work.
-
Organize Your Data: Ensure that your dataset is clean and ready for analysis. This means checking for any missing data or errors that could affect the reliability assessment. Each rater’s scores should be clearly labeled, and the data should be structured in a way that allows for easy comparison across raters.
-
Choose the Right Method: Select the appropriate statistical method to assess inter rater reliability. As mentioned earlier, the Intra Class Correlation Coefficient (ICC) is a popular choice, especially when dealing with continuous data and multiple raters. However, depending on your data type and research design, you might also consider other methods like Cohen’s Kappa for categorical data. Selecting the right method is key to accurately interpret inter rater reliability.
-
Run the Analysis: Use software tools like SPSS, R, or Python to calculate the ICC or other reliability metrics. These tools often have built-in functions or packages that simplify the process. After running the analysis, you’ll receive outputs that include reliability coefficients, which you can then interpret to determine the level of agreement among your raters.
-
Interpret the Results: Look at the reliability coefficients provided by your analysis. For instance, if you’re using ICC, check if the value falls within an acceptable range (e.g., above 0.75 for excellent reliability). This will help you decide if your data is reliable enough to support your research conclusions. By carefully assessing inter rater reliability, you ensure the robustness of your research findings and enhance the credibility of your study.
In the next section, we will explore how to test for absolute agreement using SPSS, a practical way to ensure your data is consistent and reliable.
Testing for Absolute Agreement in SPSS
Testing for absolute agreement in SPSS is a practical way to ensure your data is consistent and reliable. If you’re new to SPSS or looking to refine your skills, we’ll guide you through the steps needed to conduct this analysis effectively.
-
Prepare Your Data: Make sure your data is clean to avoid errors. Each rater’s scores should be entered correctly and clearly labeled in your dataset. This organization is crucial for running any analysis smoothly.
-
Set Up the Analysis: Open SPSS and load your dataset. Navigate to “Analyze” in the top menu, then choose “Scale” followed by “Reliability Analysis.” In this dialog box, you’ll find the option to select the type of ICC you want to use. For absolute agreement, you’ll typically choose the two-way mixed model for absolute agreement.
-
Run the Analysis: Once you’ve set up your analysis, click “OK” to run it. SPSS will process your data and provide you with an output that includes the ICC values. This output will help you interpret inter rater reliability using the Intra Class Correlation Coefficient.
-
Interpret the Results: Look at the ICC value to see if it indicates a high level of agreement (values closer to 1) or a lower level (values closer to 0). If your ICC value is high, it suggests that your raters are in strong agreement, meaning your data is reliable. If the value is low, you may need to investigate why raters are not agreeing and consider adjusting your rating criteria or training.
By testing for absolute agreement in SPSS, you can confidently ensure that your data is trustworthy and ready for further analysis. In our next section, we will discuss how to determine if the results are acceptable to conclude inter rater reliability and what this means for your research.
Concluding Inter Rater Reliability
After you have analyzed your data, the next step is to determine whether your results are acceptable to conclude inter rater reliability. This is a critical part of your research process because it informs you about the level of confidence you can have in your findings. Let’s explore how to make this determination and what it means for your study.
To begin, examine the reliability coefficients from your analysis, such as the Intra Class Correlation Coefficient (ICC), to interpret inter rater reliability. As a reminder, an ICC value closer to 1 signifies excellent agreement among raters, indicating that your ratings are consistent and reliable. Typically, an ICC greater than 0.75 suggests excellent reliability, while values between 0.60 and 0.75 indicate good reliability. If your ICC falls within these ranges, your results are generally considered acceptable to conclude inter rater reliability.
However, if your ICC value is lower, below 0.60, it’s important to reflect on the possible reasons for this and what actions you might take. Consider whether there were any inconsistencies in the rating criteria or if raters received sufficient training. Low inter rater reliability can signal the need for revisiting how raters are instructed or how the measurement process is structured. Addressing these issues can help improve consistency in future research.
Once you establish that your results are acceptable, it’s crucial to understand the implications for your research. High inter rater reliability means that the data collected is consistent across different observers, which strengthens the validity of your study’s conclusions. It ensures that your findings are based on reliable observations, making them more credible and impactful.
In conclusion, determining if your results are acceptable to conclude inter rater reliability is a vital step in ensuring the overall quality of your research. By interpreting the results correctly and addressing any discrepancies, you enhance the robustness of your study. This not only boosts your confidence in your findings but also contributes to the broader field of research by providing reliable data. With these insights, you’re well-equipped to ensure your research is based on solid and reliable data.