Multiple Poisson Distributions Explained

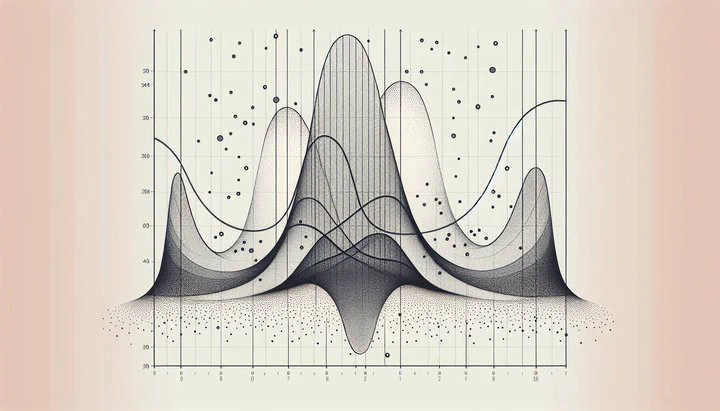
Multiple Poisson Distributions Explained
Have you ever wondered how statisticians predict random events? This is where Poisson distributions come into play. Poisson distributions help us understand how events happen over a fixed time or space. For example, they can predict the number of calls a call center gets each hour or how many emails you receive daily.
The magic of Poisson distributions is their ability to find patterns in randomness. They are often used when events occur independently, and the rate at which they happen stays the same. For instance, if you want to predict how often a server might crash in a month or how many customers might visit a store in an hour, Poisson distributions can help.
Imagine you’re running a busy hospital emergency room. You need to know how many patients to expect each hour to schedule enough doctors and nurses. By using a Poisson distribution, you can estimate the average number of patients and be ready for busy or slow times. This helps ensure timely care and efficient use of resources.
Understanding Poisson distributions is essential for anyone involved in statistical analysis. They allow us to see patterns in randomness and make informed decisions based on data. Whether you’re a student, researcher, or professional, mastering this concept can assist you in analyzing situations where events are random but occur with a known average rate. As we dive deeper into multiple Poisson distributions explained, you’ll discover how these principles extend to more complex scenarios, offering greater insights and applications.
Exploring Multiple Poisson Distributions
Now that we’ve laid the groundwork with Poisson distributions, let’s explore multiple Poisson distributions explained. You might wonder, “What exactly are multiple Poisson distributions, and how do they differ from a single Poisson distribution?” At its core, a multiple Poisson distribution involves more than one Poisson process. It’s often used when you want to model several events happening independently over the same time or space.
Think about managing not just one call center, but three. Each center gets calls at different rates, but you want to understand the overall call traffic. In this case, using multiple Poisson distributions allows you to model each center’s call rate separately and then combine them to see the bigger picture. The difference and when would I use them can be seen in scenarios like this, where multiple independent events need to be considered together.
Multiple Poisson distributions become particularly useful when the events you’re studying don’t affect each other. For example, suppose you’re examining the number of emails received across different departments of a company. Each department’s email traffic can be modeled with its own Poisson distribution, and together, they form a set of multiple Poisson distributions. This approach helps in understanding the overall communication load and aids in resource allocation.
The key to effectively using multiple Poisson distributions lies in recognizing when events are independent and occur at different rates. By understanding these nuances, you can gain deeper insights into complex systems, like tracking customer arrivals in different store sections or analyzing traffic flow through various city intersections. As we proceed, we’ll explore the different variants of multiple Poisson distributions and how they find practical applications in diverse fields, enhancing your ability to apply these tools in research and data analysis.
Variants and Practical Applications
As we continue our exploration of multiple Poisson distributions, it’s important to delve into the variants that can arise and understand their practical applications. Each variant serves a unique purpose, helping us tailor our analysis to specific situations.
One common variant is the variant of the second one, often used when dealing with hierarchical or nested events. Imagine you’re analyzing the number of website visits from different regions. Each region can be modeled with its own Poisson distribution, but when you want to assess the entire country’s web traffic, you might use this variant to combine the regional data effectively.
Another variant involves using multiple Poisson distributions to model events that have a dependency or influence between them. For instance, in a hospital scenario, you’re tracking patient visits to different departments, like the emergency room and outpatient services. If there’s a flu outbreak, patient numbers might rise in both departments simultaneously. This complex relationship can be captured using a more sophisticated variant of multiple Poisson distributions.
Practical applications of these variants are vast and varied. In retail, they help understand shopping patterns across different store locations, enabling better inventory management. In transportation, they model traffic flow across multiple routes, aiding in city planning and congestion reduction. In healthcare, they assist in predicting patient load in different hospital units, ensuring optimal staff allocation.
When choosing the right variant, consider the specifics of your data and the relationships between events. This ensures your analysis is both accurate and insightful, leading to more informed decisions. As you become familiar with these variants, you’ll find them invaluable in tackling complex statistical challenges, enhancing your ability to apply these concepts effectively in your work or studies.
Choosing the Right Distribution
Selecting the correct Poisson distribution for your analysis can seem challenging, but with a bit of guidance, you can make the right choice. Let’s break it down into simple steps so you can confidently decide which Poisson distribution to use in your work or studies.
First, consider the nature of your data. Are you dealing with a single event occurring over time or space, like the number of cars passing a checkpoint each hour? If so, a single Poisson distribution will likely suffice. However, if you’re handling multiple independent events, like calls to different departments within a company, then multiple Poisson distributions are what you need. These allow you to model each department separately and understand the overall picture.
Next, think about the relationships between your events. If the events are independent, you can use standard multiple Poisson distributions. But, if there’s a dependency, like a flu outbreak affecting several hospital departments simultaneously, you might need a variant of the second one. This variant captures dependencies and provides a more comprehensive analysis.
To help you choose, here’s a simple checklist:
- Identify if your events are independent or dependent.
- Determine if you’re analyzing one event or multiple events.
- Consider if events occur at different rates.
- Decide if you need to account for any dependencies between events.
By following these steps, you can avoid common pitfalls, like incorrectly assuming independence between events or overlooking dependencies. This ensures your analysis remains accurate and insightful.
Remember, choosing the right distribution is key to unlocking valuable insights from your data. With practice, you’ll become adept at selecting the appropriate Poisson distribution, empowering you to make data-driven decisions with confidence. As you grow more comfortable with these concepts, you’ll be ready to tackle even more complex statistical challenges in your work or studies.
Conclusion and Further Resources
In this journey through multiple Poisson distributions explained, we’ve uncovered the power of Poisson distributions in analyzing random events and their applications in various fields. From understanding the basics of Poisson distributions to exploring their multiple variants, we’ve equipped you with the tools to analyze complex scenarios with greater precision.
Remember, multiple Poisson distributions are invaluable when dealing with independent events that occur simultaneously but at different rates. They help you see the bigger picture, whether you’re managing multiple departments, predicting customer arrivals, or analyzing traffic patterns. When events are dependent or influence each other, knowing how to apply a variant of the second one can enrich your analysis and provide deeper insights.
To continue your exploration and deepen your understanding, consider diving into additional resources. Books like “Introduction to Probability Models” by Sheldon Ross offer detailed explanations and examples of Poisson processes. Online courses on platforms such as Coursera or edX can provide structured learning paths and practical exercises. Websites like StatisticalExplorer.com are great for tutorials and discussions on statistics and data science.
For those seeking community support or further discussion, subreddits like “rstats” and “AskStatistics” are excellent places to connect with peers and experts alike. Engaging with these communities can enhance your learning experience and allow you to share knowledge and insights with others.
By building on the knowledge gained here, you’ll be able to apply these concepts effectively in your work or studies, making data-driven decisions with confidence. Whether you’re a student, researcher, or professional, the world of Poisson distributions is rich with opportunities for discovery and application. Keep exploring, learning, and growing, and you’ll find yourself well-equipped to tackle the statistical challenges that come your way.