Using Non Parametric Test Mann-Whitney for Product Revenue

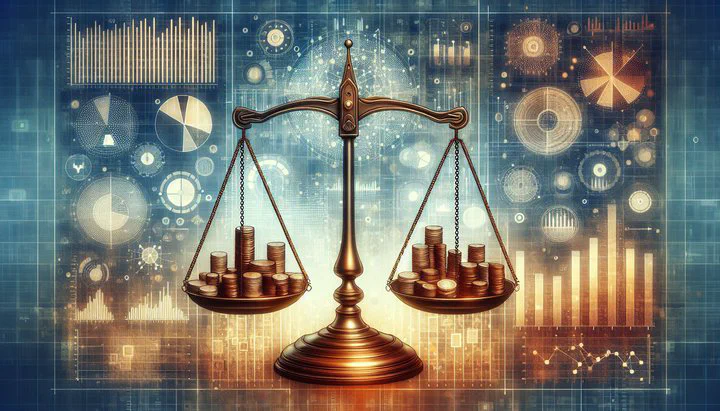
Using Non Parametric Test Mann-Whitney for Product Revenue
Introduction to Non-Parametric Tests
In statistical analysis, it’s common to encounter data that doesn’t follow a normal distribution. Have you ever wondered what to do when your data doesn’t follow the normal bell curve? That’s where non-parametric tests come to the rescue! These tests are special because they don’t need your data to fit any specific shape or follow strict rules. Unlike parametric tests, which assume your data is like a neat, normal distribution, non-parametric tests give you the freedom to analyze data that’s a bit messy or unusual.
Non-parametric tests are important when working with data that breaks the usual assumptions of parametric tests. For example, imagine you’re working with product revenue data. Often, product revenue is not normally distributed, meaning it doesn’t fit the nice, symmetrical pattern that parametric tests love. This is where non-parametric methods, such as the non parametric test Mann-Whitney, come into play.
So, when should you choose a non-parametric test over a traditional one? If your data is skewed, has outliers, or comes in ranks instead of precise numbers, non-parametric tests are your best bet. Let’s say you’re comparing two groups: maybe you’re running an AB test where the main KPI I am following is product revenue. If the revenue data looks a bit wonky and doesn’t fit the normal curve, a non-parametric test like the Mann-Whitney is a smart choice. It helps you compare the groups without worrying about those pesky distribution issues.
In summary, non-parametric tests are handy tools for your statistical toolbox. They help when your data doesn’t play by the usual rules, letting you compare groups and make informed decisions without the constraints of normality. Whether you’re a student or a data professional, knowing when and how to use these tests can make your analyses stronger and more reliable.
Understanding the Mann-Whitney Test
Now that we know non-parametric tests are great for data that doesn’t fit the usual mold, let’s dive into one of the most popular ones: the non parametric test Mann-Whitney. This test is your go-to tool when you want to compare two independent groups but can’t rely on the normal distribution. It’s like having a fair judge who doesn’t care if your data is a little quirky or unconventional.
So how does the Mann-Whitney test work? Imagine you’re comparing the sales of two different products, and your data is all over the place. You can’t just use a regular t-test because product revenue is not normally distributed. Instead, the Mann-Whitney test ranks all the sales numbers from both products together, then checks if one product tends to have higher ranks than the other. It’s like a race where you want to see not just who finishes first, but how everyone else ranks too.
Why choose the Mann-Whitney test over a parametric test like the t-test? Well, if you want to compare means between versions and n is large, but your data doesn’t meet the strict assumptions of normality, the Mann-Whitney test is perfect. It doesn’t care about extreme values (outliers) that might skew your results. This makes it robust and reliable for real-world data that doesn’t behave perfectly.
In summary, the Mann-Whitney test is a powerful tool for comparing two groups without worrying about normality. If you’re faced with the question, “Instead should I run a non parametric test (e.g Mann-Whitney)?”, remember that this test is your friend when dealing with non-normal data. Whether you’re a student analyzing research data or a professional making business decisions, the Mann-Whitney test helps you draw meaningful conclusions with confidence.
Product Revenue and Distribution Assumptions
When analyzing product revenue data, you might notice something interesting: it’s often not shaped like a perfect bell curve. In fact, product revenue is not normally distributed in many cases. This can happen because revenue data often includes outliers, like a sudden surge in sales or an unexpected downturn, which can make the data appear skewed or uneven.
So why does this matter when you’re trying to compare groups or test hypotheses? Traditional tests like the t-test need data to fit a normal distribution. But when your data doesn’t, these tests might not give you accurate results. They could mislead you into thinking there’s a difference when there isn’t, or vice versa. That’s why understanding your data’s distribution is crucial before jumping into analysis.
Let’s say you’re working on an AB test where the main KPI I am following is product revenue. If your revenue data is skewed, using a parametric test might not be your best option. Instead, you should consider a non-parametric test, like the non parametric test Mann-Whitney. This test doesn’t require the data to be normally distributed, making it perfect for situations where your revenue data is all over the place.
Remember, the shape of your data affects the type of test you should use. If you’re dealing with non-normal data and want to compare means between versions and n is large, the Mann-Whitney test could be your go-to choice. It allows you to make comparisons without worrying about the normality assumption, helping you make informed decisions based on your actual data, not just what the textbook says it should look like.
In summary, when your product revenue data doesn’t fit the normal distribution, it’s important to choose the right test. The Mann-Whitney test offers a reliable alternative, ensuring that your analysis reflects the true nature of your data. Whether you’re a student or a data analyst, understanding these distribution assumptions empowers you to choose the best tools for your research or business needs.
Applying Mann-Whitney in A/B Testing
When you’re running an AB test where the main KPI I am following is product revenue, choosing the right statistical test is crucial. If your revenue data isn’t normally distributed, the non parametric test Mann-Whitney is a great option. Let’s walk through how you can set up your A/B test and use this test to compare two groups effectively.
First, split your audience into two groups. Each group sees a different product version. Then, collect and rank the revenue data from both groups together.
Next, check the distribution of your revenue data. If you find that product revenue is not normally distributed—perhaps it’s skewed due to a few big sales or some outliers—a parametric test like the t-test might not be suitable. In this case, you want to compare means between versions and n is large, and the Mann-Whitney test is ideal because it doesn’t assume your data is normally distributed.
To apply the Mann-Whitney test, start by ranking all the revenue data from both groups together. The test then examines these ranks to determine if one group consistently has higher (or lower) revenue than the other. This method is robust against outliers, so those unusually high or low sales won’t skew your results.
Finally, look at what the results tell you. If the Mann-Whitney test shows a significant difference in ranks between the two groups, you can conclude that one version of your product page is likely generating more revenue than the other. This insight allows you to make data-driven decisions about which version to prioritize.
In summary, when conducting an A/B test with non-normal revenue data, the non parametric test Mann-Whitney helps you compare groups effectively. By choosing this test, you ensure that your analysis accurately reflects your data’s true characteristics, enabling you to make informed decisions that drive business success.
Deciding Between Parametric and Non-Parametric Tests
How do you know which test to use for your data? Choosing the right test for your data analysis can feel like a big decision. You might wonder, “Do I use a t-test, or instead should I run a non parametric test (e.g Mann-Whitney)?” Let’s explore how to make this choice based on your data and research goals.
First, consider the distribution of your data. If your data follows a normal distribution, parametric tests like the t-test are usually appropriate. They work well when you have data that’s symmetrical and bell-shaped. But, if your product revenue is not normally distributed, parametric tests might not give reliable results. In such cases, a non-parametric test, like the non parametric test Mann-Whitney, is a better choice because it doesn’t require normally distributed data.
Next, think about your sample size. With large sample sizes, both parametric and non-parametric tests can be effective. However, if you want to compare means between versions and n is large, but your data isn’t normal, the Mann-Whitney test can handle it without the strict assumptions of parametric tests. This flexibility makes non-parametric tests valuable when you’re unsure about your data’s distribution.
Also, consider your research objectives. Are you looking at rankings or medians rather than means? If so, non-parametric tests are designed to analyze these kinds of data. They are perfect for situations where your data includes outliers or is ordinal (ranked rather than measured precisely).
To sum it up, deciding between parametric and non-parametric tests depends on your data’s characteristics and what you aim to find out. If your data doesn’t meet the assumptions needed for parametric tests, or if it’s skewed and full of surprises, non-parametric tests like the Mann-Whitney are your go-to tools. By understanding these factors, you can choose the most appropriate test, ensuring your analysis is accurate and meaningful. Whether you’re a student or a seasoned analyst, knowing when to use each test empowers you to make informed, data-driven decisions.
Conclusion
In the world of data analysis, choosing the right test is key to drawing accurate conclusions. When faced with non-normal data, especially in situations like A/B testing where product revenue is the main focus, the non parametric test Mann-Whitney stands out as a reliable option. By understanding when and how to apply this test, you enhance your toolkit for making data-driven decisions. So, next time you’re working with data that doesn’t fit the usual patterns, remember the power of non-parametric tests. They can help you see through the noise and uncover the true story your data is telling.