Specific Values in Multilevel Model Analysis

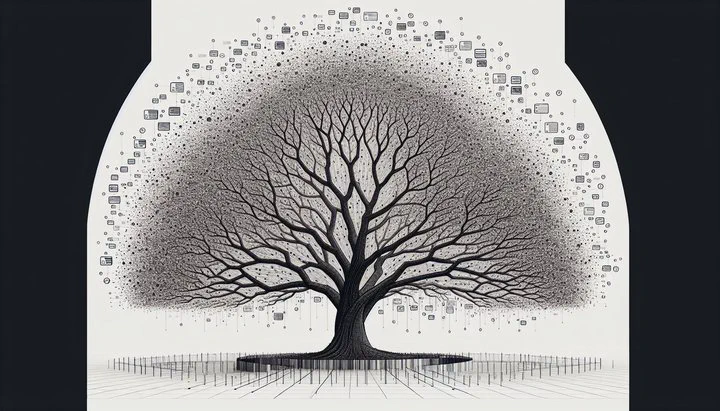
Understanding Multilevel Model Analysis
Have you ever wondered how researchers make sense of data that seems to have layers or levels, like layers of an onion? That’s where multilevel model analysis comes in! It’s a special way to look at data that has a hierarchical structure, like students in classes or patients in hospitals. This kind of analysis helps you see the bigger picture without losing the details.
Multilevel model analysis is important because it helps us understand complex data in fields like psychology, education, and social sciences. Imagine you’re studying how different teaching methods affect students’ test scores. Students are in classes, and classes are in schools. A multilevel model can analyze test scores while considering these different layers to see how much of the score differences come from individual students, classes, or schools.
One of the coolest things about multilevel models is they let you explore data that doesn’t fit neatly into single-level analyses. For instance, if you’re looking at patients’ recovery times, patients might be grouped by doctors, and doctors by hospitals. Multilevel model analysis lets you consider these layers, giving you a deeper understanding of how each level affects your results.
In short, multilevel model analysis is a powerful tool for researchers. It helps make sense of complex, layered data and draws out insights that simpler methods might miss. As you dive into analyzing specific values in multilevel model analysis, remember this approach is all about uncovering the story behind the numbers—layer by layer.
Working with Specific Values of Categorical Variables
When diving into multilevel model analysis, you’ll often need to work with categorical variables. What are these? They’re types of data divided into groups or categories, like colors (red, blue, green) or pets (cats, dogs, birds). In research, these might be different teaching methods or patient treatment types.
Understanding specific values of two categorical variables is really important for making precise analyses. Imagine two teaching methods (Method A and Method B) and two school types (Public and Private). If you want to see how each teaching method performs in each school type, you’ll need to analyze data for specific values—like Method A in Public schools and Method B in Private schools.
However, working with two categorical variables can be tricky. You might face challenges like too many combinations to analyze or not enough data for each category pair. That’s why specifying the right values is key. By focusing on specific values in multilevel model analysis, you target your analysis to look at the exact scenarios you’re interested in.
To tackle these challenges, it’s helpful to use examples. Let’s say you’re studying how different diets affect people’s health across age groups. Your categorical variables are ‘Diet Type’ (Vegan or Mediterranean) and ‘Age Group’ (Teens or Adults). By analyzing specific values—like Vegan diets for Teens—you can find out which combinations lead to better health outcomes.
In summary, when you specify the value of CATA and CATB in your multilevel model analyses, you conduct more meaningful and targeted research. This step is crucial for capturing the complexity of real-world data, leading to insights that can improve decision-making in your field.
Running Separate lme or lme4 Analyses
When you’re working with multilevel model analysis, sometimes you need to dig deeper into your data. This is where running separate analyses using tools like lme or lme4 can really help. These tools are powerful for analyzing data with a hierarchical structure, and they let you focus on specific values within your dataset.
Imagine you have data with two categorical variables, like different teaching methods and school types. If you want to understand how each teaching method performs in each school type, you might need to run four separate lme or lme4 analyses. This means you’ll run one analysis for each combination of the two categorical variables. For example, one analysis for Method A in Public schools, another for Method A in Private schools, and so on. By doing this, you see the effects of each variable combination clearly.
Running these separate analyses can seem a bit daunting, but it’s manageable with a step-by-step approach. First, install the lme4 package if you’re using R. Load your data and set up your model. Here’s a simple code snippet:
library(lme4)
# Assuming you have a dataset called 'data' with columns 'TeachingMethod', 'SchoolType', and 'Score'
model <- lmer(Score ~ TeachingMethod + (1|SchoolType), data = data)
summary(model)
In this example, Score
is the outcome you’re interested in, while TeachingMethod
and SchoolType
are your categorical variables. By running separate models for each combination, you gain a deeper understanding of how they interact.
The purpose of running separate analyses is to untangle the effects of your categorical variables. This helps you focus on the specific values that matter most to your research question. By isolating these effects, you can make more precise conclusions about your data.
In conclusion, using lme or lme4 for separate analyses is a vital skill in multilevel modeling. It allows you to analyze data for specific values, giving you the tools for informed, detailed interpretations. Embrace this approach to uncover the unique stories your data has to tell!
Specifying Values in Multilevel Models
In multilevel model analysis, being able to specify values of categorical variables is like having a magnifying glass to zero in on details that matter most. When you specify the value of CATA and CATB, you’re making strategic choices that can influence your study’s outcomes.
Let’s break this down. Imagine your categorical variables, CATA and CATB, represent different teaching methods and student demographics. To analyze how a particular teaching method works for a specific student group, you need to specify the values of these variables. This means deciding exactly which method and student group you’re going to look at together, tailoring your analysis to uncover specific insights.
The process can be streamlined using tools like R. You can use packages like lme4 to set specific values in your models. Here’s a simple way:
# Load lme4 package
library(lme4)
# Specify the values for CATA and CATB
subset_data <- data[data$TeachingMethod == "Method A" & data$StudentGroup == "Group 1", ]
# Run the model on the specified subset
model <- lmer(Score ~ (1|School), data = subset_data)
summary(model)
In this example, we specify we want to look at “Method A” for “Group 1” students. This helps us analyze data for specific values, allowing us to see how this combination affects the outcome, like students’ test scores.
When you specify these values correctly, your results are more precise and relevant. You can handle complex questions like, “Does Method A work better for Group 1 than for Group 2?” By focusing on specific values, you’re enhancing your analysis’s quality, allowing for nuanced interpretations.
In summary, specifying values in multilevel models is essential for conducting precise and meaningful analyses. By using tools like R and specifying CATA and CATB values, you gain the power to explore intricate data details. This practice improves your analytical skills, leading to deeper insights and smarter decision-making.
Applications and Insights
Analyzing specific values in multilevel model analysis is more than just a technical exercise—it’s a way to unlock insights with real-world applications. Whether in social sciences, education, or healthcare, understanding how different factors interact at various levels can drive informed decisions and solutions.
Consider education research. To understand teaching methods’ impact on student performance across socioeconomic backgrounds, you examine specific values of two categorical variables—such as “Teaching Method” and “Socioeconomic Status.” You discover how a teaching strategy might be effective for students from lower-income families. This targeted analysis helps educators tailor approaches to meet diverse needs, improving educational outcomes.
In healthcare, multilevel models analyze patient data across hospitals and treatment types. By specifying the value of treatment type (CATA) and hospital type (CATB), researchers identify which protocols work best in different settings. For instance, a study might show a medication is more effective in community hospitals than urban centers, guiding treatment plans and patient care.
Insights from specific values in multilevel models are useful for researchers and policymakers. In economics, understanding how policies affect demographics can lead to equitable strategies. By running separate analyses, like run four separate lme or lme4 analyses, economists assess a policy’s impact on income groups and regions, ensuring interventions are fair and impactful.
In conclusion, applying multilevel model analysis to analyze data for specific values provides a nuanced understanding of complex issues. This method delves into your data’s dynamics, offering insights to inform strategies, improve practices, and support evidence-based decision-making. Embrace this approach to make a meaningful difference in your study or work, leading to better outcomes and innovations.