Using Unpaired T-Test for Training Evaluation

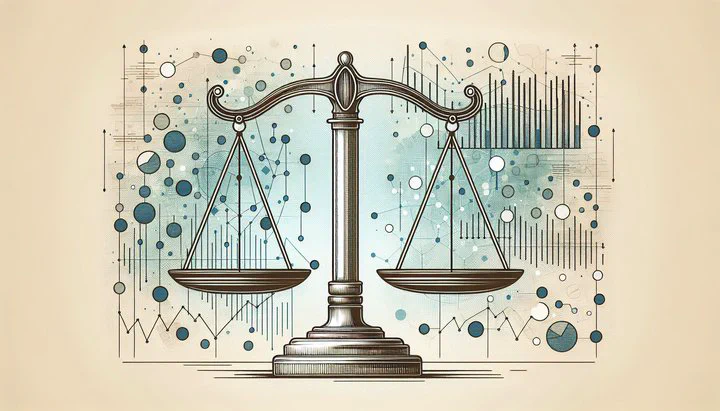
Using Unpaired T-Test for Training Evaluation
Welcome to the world of statistics, where we use numbers to tell stories and make decisions. Today, we’ll explore using the unpaired t-test for training evaluation, a powerful tool used in statistical analysis. This test helps us understand if there is a meaningful difference between two groups. It’s especially useful when evaluating training programs to see if the training made a difference.
So, what exactly is an unpaired t-test? It’s a statistical method used to compare the averages (or means) of two independent groups. Imagine you have two classes of students who took different training sessions. You want to know if one group learned more than the other. The unpaired t-test helps you find out if there is a statistically significant difference between the knowledge of groups. This means it checks if the difference in scores is likely due to the training, rather than just random chance.
Think of the unpaired t-test as a magnifying glass, helping us clearly see if training really makes a difference. When you apply this test, it tells you whether the difference in scores between the groups is big enough to be important. In statistical terms, this is called “statistical significance.” By using the unpaired t-test, you can evaluate if the training program had a real impact or if the results could have happened by accident.
In the following sections, we’ll dive deeper into how the unpaired t-test works, why statistical significance matters, and how you can use this test to compare training outcomes. Whether you’re a student, a researcher, or a data analyst, understanding the unpaired t-test will empower you to make informed decisions about training effectiveness. Let’s unlock the potential of data to improve training programs and enhance learning experiences!
Understanding Statistical Significance
When using the unpaired t-test for training evaluation, a key concept to grasp is statistical significance. But what does this term really mean, and why is it important? In simple terms, statistical significance helps us determine if the differences we observe between two groups are genuine or just happened by chance.
Imagine you’ve tested two groups of students who attended different training programs. If one group scores higher than the other, you might wonder if the difference is meaningful. This is where statistical significance comes in. It tells us if there is a statistically significant difference between the knowledge of groups. In other words, it helps us find out if there is any statistically significant difference that can be attributed to the training rather than random variation.
So, how does the unpaired t-test help in determining statistical significance? Here’s a simple explanation: the test calculates a p-value, which is a number that tells you how likely it is that the observed differences are due to chance. If the p-value is low, it means the training likely caused the difference, not just luck.
Understanding statistical significance is crucial when evaluating the effectiveness of a training program. It helps you make informed decisions by providing evidence that the training made a real impact. Without considering statistical significance, you might mistakenly conclude that a training program was successful or unsuccessful based solely on observed differences, which might not be reliable. By focusing on statistical significance, you ensure your conclusions are backed by solid evidence.
In the next section, we’ll explore how to use the unpaired t-test to compare the pre-test and post-test scores of different groups. This will further illustrate the importance of statistical significance in assessing training outcomes. Let’s continue to use data to uncover insights and improve training effectiveness!
Comparing Pre-Test and Post-Test Scores
When you’re evaluating a training program, one of the most insightful methods is to compare the pre-test score of one group with the post-test score of another. This approach helps you measure the impact the training had on participants’ knowledge. The unpaired t-test is a handy tool for this task.
Imagine you have two groups of students. Group A took a pre-test before the training, and Group B took a post-test after the training. You want to see if the training led to a significant improvement in scores. By using the unpaired t-test, you can determine if the difference in scores between these two groups is truly significant or just a result of random chance.
Here’s how it works:
- Step 1: Collect the pre-test scores for Group A and the post-test scores for Group B.
- Step 2: Use the unpaired t-test to compare these scores.
- Step 3: Look at the p-value to see if there’s a statistically significant difference between the knowledge of the groups after the training.
If the test shows a significant difference, it suggests that the training had a positive effect on the learners’ knowledge.
Let’s look at an example. Suppose Group A scored an average of 70 on the pre-test, while Group B scored an average of 85 on the post-test. You run the unpaired t-test, and it gives you a p-value of 0.03. Since this p-value is less than 0.05, you can conclude that the improvement in scores is statistically significant. This means the training likely led to the increase in scores, and it wasn’t just a random occurrence.
By comparing pre-test and post-test scores with the unpaired t-test, you gain valuable insights into the effectiveness of your training program. This method allows you to confidently assess whether the training achieved its goals and is indicative of the quality of the training. In the next section, we’ll explore how these results can reflect the overall quality of the training program. Let’s continue to use data to enhance our understanding and improve learning outcomes!
Quality Indicators of Training Programs
Evaluating the effectiveness of a training program is crucial to ensure it meets its learning objectives. The results of using an unpaired t-test for training evaluation can be indicative of the quality of the training. But how can you interpret these results to assess training quality effectively?
When the unpaired t-test shows a statistically significant difference between the knowledge of groups, it suggests that the training had a meaningful impact on participants. This significant difference is a key quality indicator, as it demonstrates that the training provided valuable learning experiences that led to improved knowledge or skills.
To further assess the quality of a training program, consider the magnitude of the difference in scores. A large and statistically significant difference often indicates a highly effective training. Conversely, a small difference, even if statistically significant, might suggest that while the training had an impact, it may not be substantial enough to meet your goals.
It’s also important to see if you get the same results every time. If multiple training sessions consistently show statistically significant improvements, this strengthens the case for the program’s effectiveness. On the other hand, inconsistent results across different sessions could signal variability in the training delivery or content, which may need to be addressed.
Finally, consider the practical implications of the findings. Beyond statistical significance, a training program should lead to real-world improvements in performance or knowledge. Discussing these practical outcomes with stakeholders helps ensure that the training is not only statistically effective but also meaningful in the context of the learners’ needs and the organization’s goals.
By using the unpaired t-test, you can gain a comprehensive understanding of how effective a training program is. These insights can guide future improvements and help design even more impactful learning experiences. In the next section, we’ll explore a real-world example of how the unpaired t-test is applied in training evaluation, bringing these concepts to life. Let’s continue to harness the power of data to enhance training programs and achieve better outcomes!
Real-World Example and Application
Let’s dive into a real-world example to see how using the unpaired t-test for training evaluation can make a difference. Imagine a company wants to improve its employee productivity through a new training program. They decide to evaluate the program’s effectiveness by comparing two groups of employees.
Group A hasn’t taken the training yet and is given a pre-test to measure their current performance. Group B completes the training and takes a post-test afterward. The company wants to see if there’s a significant improvement in Group B’s performance compared to Group A’s pre-test scores.
The data shows that Group A scored an average of 60 points on the pre-test, while Group B scored an average of 80 points on the post-test. The company uses the unpaired t-test to compare these scores and find out if there is any statistically significant difference between the two groups.
After running the test, they find a p-value of 0.02. This p-value is less than 0.05, which means the difference in scores is statistically significant. The training likely caused the improvement, indicating the program was effective. This result is indicative of the quality of the training, showing it successfully enhanced the employees’ skills.
Beyond the numbers, the company also considers the practical impact. Employees report feeling more confident and productive, which aligns with the test results. This combination of statistical and real-world evidence confirms the training’s success.
By using the unpaired t-test, the company gains valuable insights into their training program’s effectiveness. They can now make informed decisions about future training initiatives and continue to improve their employees’ performance. This example illustrates how data-driven evaluations can lead to better outcomes and help organizations achieve their goals.
Conclusion
Using the unpaired t-test for training evaluation provides a clear, evidence-based method to assess training effectiveness. By understanding statistical significance and comparing pre-test and post-test scores, you can determine if your training programs make a real difference. Whether you’re a student, researcher, or data analyst, these skills will help you make informed decisions and improve learning experiences. Now, why not try using an unpaired t-test with your own data and see what insights you can uncover?